A.I. & Psychology
Introduction
To incorporate AI-related content into a psychology course, in particular ones that cover psychopathology (treatment, diagnosis), two modules are presented in this portfolio. Readings may be assigned from the reference list provided at the end.
The content regarding AI in psychology focuses, in part, on the use of AI-based algorithms and applications for psychological research and disease detection using publicly available clinical data. AI can be used for the analysis of epidemiological data, including social media data, for understanding mental health conditions, for disease detection, and for estimating risk of mental illness. This refers to the content of Module 1.
Another way in which AI and psychology intersect is the use of AI-assisted therapy, whether on its own or in addition to (human) therapist-led treatment. This can include internet-based cognitive behavioural therapy, mindfulness and wellbeing apps, artificial companions, virtual reality (VR) applications, therapeutic computer games, and chatbots. This refers to the content of Module 2.
Module 1 – AI & Mental Health: Research & Detection
Detecting mental illness using social media data and online behaviour lies at the intersection of computational linguistics and clinical psychology. The use of AI and machine learning using data collected from social media users has accelerated the creation of predictive tools that can be used to evaluate the risk of developing or detecting the presence of mental health disorders. Researchers have primarily focused on the following mental health disorders: mood disorders, eating disorders, schizophrenia and psychosis, and suicidal ideation (Chancellor & Choudhury, 2020).
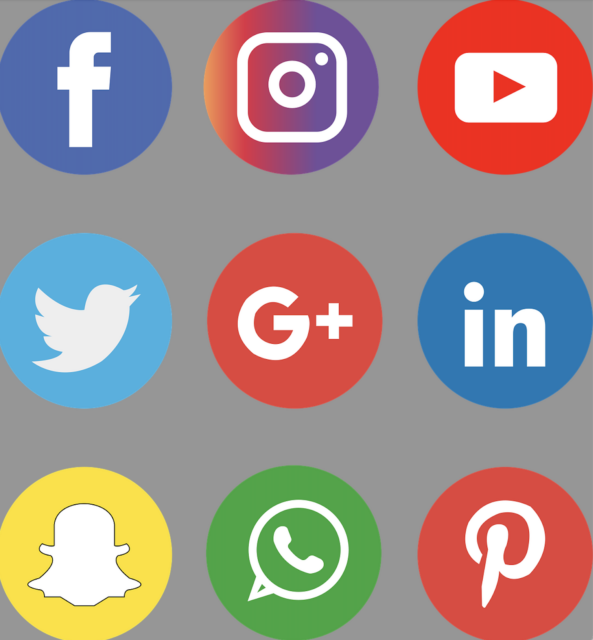
Recent studies have analyzed publicly available user data on social media platforms, such as Twitter (Amir et al., 2019; Joshi & Patwardhan, 2020; Wang et al., 2021), and Reddit (Jiang et al., 2021; Uban et al., 2021) to test whether AI can predict the risk of developing a mental health disorder, or detecting the presence of one. The consensus is that yes, the data can reveal mental health risk.
However, ethical concerns have been raised about the accuracy, reliability, and validity of these methods (Orr et al., 2022). In addition, this leads to other questions, such as, what are the risks/benefits? What are the short-term vs. long-term implications? Does disease detection streamline access to intervention, or timely mental health care? How should social media users be notified (if at all) of their predicted risk?
An open-source directory of data sets intended to facilitate research in this field was compiled and made available by Harrigian et al. (2021), as well as a data set comprised of mental health keywords used on Twitter tweets (Harrigian, 2020). Links to these are provided at the end of this portfolio.
Given that social media use and having an active online presence is a large part of contemporary culture, it is expected that many students are users of multiple such platforms. This topic therefore lends itself well to classroom discussions, self-reflections, as well as projects/essays, for students enrolled in a psychology course.
Module 2 – AI & Mental Health: Treatment
In 2020, much of the world was in lockdown in response to the Covid-19 pandemic. This invariably led to a shift in how people receive mental health care: while lockdown restrictions were in place, synchronous telemedicine replaced in-person treatment. Although the pivot to providing and receiving treatment online avoided major treatment disruptions, the fact remains the the mental healthcare system in many countries is over-burdened, understaffed, and not accessible to everyone who needs care (Johnson et al., 2021). Moreover, the demand for mental healthcare grew exponentially during the pandemic (Aknin et al., 2022; Singu, 2022), and it is clear the system needs improvement (Aknin et al., 2022; Moreno et al., 2020). Perhaps the solution lies with AI?
The use of synchronous and asynchronous AI-assisted therapy (virtual reality (VR), mixed reality (MR), augmented reality (AR), chatbots, apps, etc.) was being used well before the pandemic, however, since 2020 the use of AI-assisted therapy has increased substantially (Carlbring et al., 2023).
Synchronous AI-assisted psychotherapy using VR or MR/AR is often used to treat anxiety disorders, phobias, stress and posttraumatic stress disorders, eating disorders, neurological rehabilitation, pain management, even psychosis (Ma et al,. 2021). It can also be used to develop empathy (Shashkevich, 2018). However, its strengths are also its weaknesses: Ma et al. (2021) point out that the efficacy of VR/MR/AR may be diminished because patients know the situation is not “real,” conversely, some patients may feel safer when not exposed to realistic scenarios that normally cause their distress. In short, this form of AI-assisted therapy is still developing and needs standardization, and access to treatment may still pose a barrier.
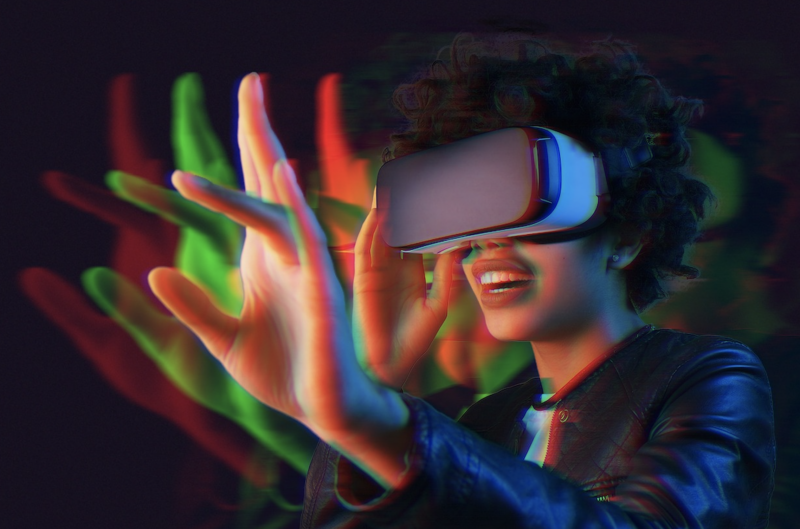
A possible solution to overcoming accessibility barriers may lie in the hands of asynchronous AI-assisted therapy. In fact, mental health chatbots, or chat therapists, have existed since the 1960s. The most famous of these – ELIZA (http://psych.fullerton.edu/mbirnbaum/psych101/eliza.htm) – was developed to generate human-like responses to natural language input in a psychotherapeutic setting (Weizenbaum, 1966). Chatbots rely on recognizing scripts or patterns of language in order to generate responses (Coheur, 2020), but can they reliably mimic humans and appear intelligent? In other words, can they pass the Turing test (Oppy & Dowe, 2021)?
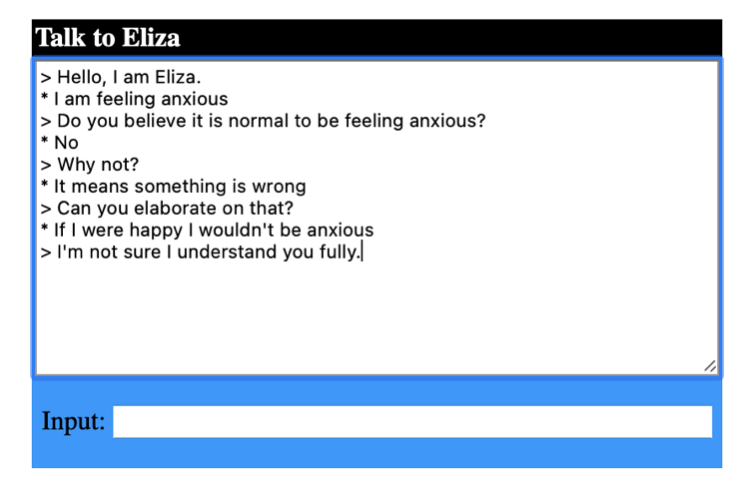
Researchers found that in general, patients do have favourable views of chat therapists, however chatbot scripts still need refining in order to be able to offer personalized care to the level of a human psychotherapist (Abd-Alrazaq et al., 2021). Although patients recognize that chat therapists are not human, they can still develop trust towards them (Bram, 2022; Darcy et al., 2021), and trust is essential for the efficacy of in-person psychotherapy (Li et al., 2021).
The company behind the WoeBot app (https://woebothealth.com/) argues that its brand of AI-assisted psychotherapy is worth patients’ money since it is: 1) free, 2) available 24/7, and 3) can be accessed without a referral or prescription. However, certain user data is collected (as outlined in their privacy policy). The ethical implications behind this must be considered: in short, does the end justify the means?
Epidemiological studies estimate that up to 50% of people will receive treatment for a mental health disorder at some point in their lives (Kessler et al., 1994, as cited in Pedersen et al., 2014), which makes AI-assisted therapy a pertinent and relevant topic for students enrolled in a psychology course, given the current state of the mental healthcare system.
Coursework – Option 1: Classroom discussion
The instructor prepares a class discussion, which may include using of the following prompts or questions.
Module 1 discussion can include:
- What are the risks of using AI in disease detection?
- What are the benefits of using AI in disease detection?
- What makes a good tool for detecting or assessing risk of disease?
- Can these detection methods be relied on to accurately and reliably assess the presence or risk of disease?
- How can these detection methods be improved?
Module 2 discussion can include:
- What are the risks of using AI-assisted psychotherapy?
- What are the benefits of using AI-assisted psychotherapy?
- What makes a good AI-assisted psychotherapy tool for treating disease?
- Can these AI-assisted psychotherapies be relied on to accurately and reliably treat disease?
- How can these AI-assisted psychotherapies be improved?
Coursework – Option 2: Self-reflection
Students may select or be assigned an issue from the relevant module and write a personal reflection.
Module 1 self-reflection example:
- Looking back at your social media use, would you feel comfortable if a researcher were to use your data to train an AI mental health detection tool?
- Would your answer change if you consented to being part of research study?
- Do you believe that this type of research will lead to improvements in mental health treatment, why or why not?
Module 2 self-reflection example:
- Do you think AI-assisted psychotherapy can address the shortcomings of the mental healthcare system? Why or why not?
- What are the advantages and disadvantages of synchronous vs. asynchronous psychotherapy?
- Do you believe AI will replace human psychotherapists, why or why not?
Coursework – Option 3: Project or term paper
In this assignment, students are responsible for developing a project or writing a term paper that addresses the use of AI in the field of psychotherapy. These include, but are not limited to:
- Develop a mental health app specific to to Cégep students
- Develop a solution for improving access to mental healthcare using AI to, for example:
- diagnose a mental health disorder
- reduce wait times for a psychotherapist
- streamline treatment/interventions across patients’ medical field
- Test the accuracy, reliability, and validity of AI to detect mental illness using publicly available clinical datasets, or using synthetically generated data
- Design an experiment to test the efficacy of AI-assisted psychotherapies
Links to Datasets
American Psychological Association. (2008). Links to datasets and repositories [Data sets]. APA. https://www.apa.org/research/responsible/data-links
Harrigian, K. (2020). Mental health keywords: Keywords and phrases that can be used for identifying mental-health-related conversation on Twitter [Data set]. GitHub. https://github.com/kharrigian/mental-health-keywords
Harrigian, K. (2021). Mental health datasets: An evolving list of electronic media data sets used to model mental-health status [Data set]. GitHub. https://github.com/kharrigian/mental-health-datasets
MOSTLY AI. (n.d.). Generate synthetic data. https://mostly.ai/synthetic-data-platform/generate-synthetic-data
Open Psychometrics Project (2019). Raw data from online personality tests [Data sets]. Open Psychometrics. https://openpsychometrics.org/_rawdata/
Substance Abuse & Mental Health Data Archive. (n.d.). Home. https://www.datafiles.samhsa.gov/
References
Abd-Alrazaq, A. A., Alajlani, M., Ali, N., Denecke, K., Bewick, B. M., & House, M. (2021). Perceptions and opinions of patients about mental health chatbots: Scoping review. Journal of Medical Internet Research, 23(1), e17828. 10.2196/17828
Aknin, L. B., De Neve, J.-E., Dunn, E. W., Fancourt, D. E., Goldberg, E., Helliwell, J. F., Jones, S. P., Karam, E., Layard, R., Lyubomirsky, S., Rzepa, A., Saxena, S., Thornton, E. M., VanderWeele, T. J., Whillans, A. V., Zaki, J., Karadag, O., & Ben Amor, Y. (2022). Mental health during the first year of the COVID-19 pandemic: A review and recommendations for moving forward. Perspectives on Psychological Science, 17(4), 915-936. 10.1177/17456916211029964
Amir, S., Dredze, M., & Ayers, J. W. (2019). Mental health surveillance over social media with digital cohorts. In K. Niederhoffer, K. Hollingshead, P. Resnik, R. Resnik, & K. Loveys (Eds.), Proceedings of the Sixth Workshop on Computational Linguistics and Clinical Psychology: From Keyboard to Clinic (pp. 114-120). 10.18653/v1/W19-3013
Bram, B. (2022, September 27). Opinion: My therapist, the robot: My year with WoeBot, an AI chat therapist. New York Times. https://www.nytimes.com/2022/09/27/opinion/chatbot-therapy-mental-health.html
Carlbring, P., Hadjistavropoulos, H., Kleiboer, A., & Andersson, G. (2023). A new era in internet interventions: The advent of Chat-GPT and AI-assisted therapist guidance. Internet Interventions, 32, 100621. 10.1016/j.invent.2023.100621
Chancellor, S., & Choudhury, M. D. (2020). Methods in predictive techniques for mental health status on social media: A critical review. NPJ Digital Medicine, 3(43). 10.1038/s41746-020-0233-7
Coheur, L. A. (2020). From ELIZA to Siri and beyond. In M.-J. Lesot, S. Vieira, M. Z. Reformat, J. P. Carvalho, A. Wilbik, B. Bouchon-Meunier, & R. R. Yager (Eds.), Communications in Computer and Information Science (CCIS) 1237, (pp. 29-41). 10.1007/978-3-030-50146-4_3
Darcy, A., Daniels, J., Salinger, D., Wicks, P., & Robinson, A. (2021). Evidence of human-level bonds established with a digital conversational agent: Cross-sectional, retrospective observational study. JMIR Formative Research, 5(5), e27868. 10.2196/27868
Harrigian, K., Aguirre, C., & Dredze, M. (2021). On the state of social media data for mental health research. In N. Goharian, P. Resnik, A. Yates, M. Ireland, K. Niederhoffer, & R. Resnik (Eds.), Proceedings of the Seventh Workshop on Computational Linguistics and Clinical Psychology: Improving Access (pp. 15-24). 10.48550/arXiv.2011.05233
Jiang, Z., Zomick, J., Levitan, S. I., Serper, M., & Hirschberg, J. (2021). Automatic detection and prediction of psychiatric hospitalizations from social media posts. In N. Goharian, P. Resnik, A. Yates, M. Ireland, K. Niederhoffer, & R. Resnik (Eds.), Proceedings of the Seventh Workshop on Computational Linguistics and Clinical Psychology: Improving Access (pp. 116-121). 10.18653/v1/2021.clpsych-1.14
Johnson, S., Dalton-Locke, C., San Juan, N. V., Foye, U., Oram, S., Papamichail, A., Landau, S., Olive, R. R., Jeynes, T., Shah, P., Sheridan Rains, L., Lloyd-Evans, B., Carr, S., Killaspy, H., Gillard, S., Simpson, A., & The Covid-19 Mental Health Policy Research Unit Group. (2021). Impact on mental health care and on mental health service users of the COVID-19 pandemic: a mixed methods survey of UK mental health care staff. Social Psychiatry and Psychiatric Epidemiology, 56, 25-27. 10.1007/s00127-020-01927-4
Joshi, D., & Patwardhan, M. (2020). An analysis of mental health of social media users using unsupervised approach. Computers in Human Behavior Reports, 2(100036), 1-9. 10.1016/j.chbr.2020.100036
Li, E., Midgley, N., Luyten, P., & Campbell, C. (2021). Therapeutic settings and beyond: A task analysis of re-establishing epistemic trust in psychotherapy. BMJ Open, 11(Suppl. 1), A8. 10.1136/bmjopen-2021-QHRN.21
Ma, L., Mor, S., Anderson, P. L., Baños, C., Bouchard, S., Cárdenas-López, G., Donker, T., Fernández-Álvarez, J., Lindner, P., Mühlberger, A., Powers, M. B., Quero, S., Rothbaum, B., Widerhold., B. K., & Carlbring, P. (2021). Integrating virtual realities and psychotherapy: SWOT analysis on VR and MR based treatments of anxiety and stress-related disorders. Cognitive Behaviour Therapy, 50(6), 509-526. 0.1080/16506073.2021.1939410
Moreno, C., Wykes, T., Galderisi, S., Nordentoft, M., Crossley, N., Jones, N., Cannon, M., Correll, C. U., Byrne, L., Carr, S., Chen, E. Y. H., Gorwood, P., Johnson, S., Kärkkäinen, H., Krystal, J. H., Lee, J., Lieberman, J., López-Jaramillo, C., Männikkö, M., Phillips, M. R., Uchida, H., Vieta, E., Vita, A., & Arango, C. (2020). How mental health care should change as a consequence of the COVID-19 pandemic. The Lancet: Psychiatry, 7(9), 813-824. 10.1016/S2215-0366(20)30307-2
Oppy, G., & Dowe, D. (2021). The Turing test. In E. L. Zlata (Ed.), The Stanford Encyclopedia of Philosophy (Winter 2021 edition). https://plato.stanford.edu/entries/turing-test/
Orr, M. P., van Kessel, K., & Parry, D. (2022). The ethical role of computational linguistics in digital psychological formulation and suicide prevention. In A. Zirikly, D. Atzil-Slonim, M. Liakata, S. Bedrick, B. Desmet, M. Ireland, A. Lee, S. MacAvaney, M. Purver, R. Resnik, & A. Yates (Eds.), Proceedings of the Eighth Workshop on Computational Linguistics and Clinical Psychology (pp. 17-29). 10.18653/v1/2022.clpsych-1.2
Pedersen, C. B., Mors, O., & Bertelsen, A. (2014). A comprehensive nationwide study of the incidence rate and lifetime risk for treated mental disorders. JAMA Psychiatry, 71(5), 573-581. 10.1001/jamapsychiatry.2014.16
Shashkevich, S. (2018, October 17). Virtual reality can help make people more compassionate compared to other media, new Stanford study finds. Stanford News. https://news.stanford.edu/2018/10/17/virtual-reality-can-help-make-people-empathetic/
Singu, S. (2022). Analysis of mental health during COVID-19 pandemic. International Journal of Sustainable Development in Computing Science, 4(3), 41-50.
Uban, A. S., Chulvi, B., & Rosso, P. (2021). Understanding patterns of anorexia manifestations in social media data with deep learning. In N. Goharian, P. Resnik, A. Yates, M. Ireland, K. Niederhoffer, & R. Resnik (Eds.), Proceedings of the Seventh Workshop on Computational Linguistics and Clinical Psychology: Improving Access (pp. 224-236). 10.18653/v1/2021.clpsych-1.24
Wang, N., Luo, F., Shivtare, Y., Badal, V., Subbalakshmi, K. P., Chandramouli, R., & Lee, E. (2021). Learning models for suicide prediction from social media posts. In N. Goharian, P. Resnik, A. Yates, M. Ireland, K. Niederhoffer, & R. Resnik (Eds.), Proceedings of the Seventh Workshop on Computational Linguistics and Clinical Psychology: Improving Access (pp. 87-92). 10.18653/v1/2021.clpsych-1.9
Weizenbaum, J. (1966). ELIZA: A computer program for the study of natural language communication between man and machine. Computational Linguistics, 9(1), 36-45.