Algorithmic Policing
My objective as part of the Dawson AI teaching COP was to translate some of the content developed as part of my PhD research on Algorithmic Policing into usable material for social science CÉGEP courses. I developed pedagogical material on this theme that can be applied in social science and humanities courses. As my research background is in political sociology, much material was constructed with Political Science and Sociology courses in mind (e.g., Introduction to Political Science 385-101-DW, Canadian Democracy 385-306-DW, Individuals and Society 387-101-DW, Sociology of Law 387-219-DW, Social Deviance 387-311-DW). Still, as both AI and policing are important topics in contemporary debates, my hope is that it might spark the interest of instructors from other disciplines. Second, I developed an Integrative Seminar (300-308-DW) dedicated to the study of the social impact of AI. Finally, this portfolio includes introduction modules and activities dedicated to Research Methods (300-300-DW) sections. Both the IS and RM content are sparkled with Algorithmic Policing content.
Algorithmic Policing Module
As part of this teaching COP on AI, I developed an introduction module on Algorithmic Policing. This material was constructed with Political Science and Sociology courses in mind, but I hope it can be of use for instructors in other disciplines. Both AI and policing raise important questions which benefit from as many perspectives as possible. The module is built around this PPT, which includes the following:
- Definitions of AI, Policing, and Algorithmic Policing
- Examples of algorithmic policing in Canada
- Introductions to key dimensions of Algorithmic Policing debates, notably Bias, Privacy, and Regulation

To introduce themes such as Facial Recognition or Automatic License Plate Readers, some professors might find it useful to use Google Quick, Draw! to illustrate how object recognition works. This first gives a real-life example of machine learning. In addition, once you get to later in the presentation and discuss algorithmic biases, it helps explain the role of human in feeding data to algorithms. The algorithm does not know any better than what humans tell it to be the truth. For instance, if all students started to draw squares when asked to draw boomerangs, eventually the Google algorithm will come to believe boomerangs are square.
Many readings can accompany this module. Two short texts by Elizabeth E. Joh do a great job at introducing the subject of AI and Policing. For a more detailed analysis, Joh’s article on the undue influence of surveillance technology companies on policing is a must read. This would work especially well in Law, Society, and Justice programs. If you are interested in the issue of surveillance and/or Body Cameras, I recommend this article.
Often time, students tend to assume algorithmic policing is reserved to China and the USA. To resolve this misperception, I assigned parts of the CitizenLab report on algorithmic policing technologies uses in Canada as of 2020. The Lab’s FAQ page also does a really good job at defining technologies such as Predictive Policing, ALPR, social media surveillance software, and facial recognition.
On the issue of Bias, the Conversation serves as a great contextualizing piece, which also touches on privacy. In addition, Julia Angwin, Jeff Larson, Surya Mattu and Lauren Kirchner’s ProPublica report on bail hearing risk assessment algorithms lead to great conversations in class. Granted, bail hearing is separate from policing, but it is still related to the criminal justice system. I also recommend this podcast episode from A.I. Nation. For students that are visual learners, the Wall Street Journal has a series of videos on Surveillance, from New Orleans to China.
For a condensed list of resources on Algorithmic Policing, please refer to the Law Enforcement document in the section titled “Moodle Folders: Thematic Description and Condensed List of Resources.” On the specific theme of Predictive Policing, please refer to the last section of this portfolio on Research Methods.
Integrative Seminar on the Social Impact of AI
The Integrative Seminar is the final program requirement for social science students. An IS project that excites students can have a lasting influence on them. My IS research on Oxfam Québec is one of the few projects I remember from my time as a CÉGEP student, way back when students did not have cellphones and we had to go in person to the Grande Bibliothèque to find resources. IS projects are aimed at giving students the tools and confidence to successfully transition into university programs, and to leverage various social science disciplines to increase their understanding of a specific phenomenon.
In the Integrative Seminar on “Contemporary Threats to Liberal Democracy: Artificial Intelligence,” students are asked to produce a university level paper on an AI theme, using at least three social science disciplines. The social impact of AI was a particularly well suited theme for an interdisciplinary course such as IS. Studying this theme from one disciplinary perspective gives only a partial understanding of the range of disturbances caused by AI. Algorithms impact humans in multiple ways, from the means of production in our society to the way we interact with each other. This IS theme therefore allowed to naturally address the linkages and differences among social sciences, as each discipline approaches the study of AI with its own theories, concepts, and lenses of analysis. As an emergent field of study, the social impact of AI has not yet fully developed interdisciplinary consensus on terminologies and concepts. This meant students had to look back at their disciplinary courses to widen their keywords in search engines.
Since AI might be an imposing topic for many¾there is so much to study!¾students were given a list of non-binding potential themes (4) and fields (3) to inspire their topic selection. Each theme and topic had a dedicated Moodle folder with a list of 5-20 resources and references to help them choose what to work on, but also guide them in their search for pertinent sources. These included links to academic articles that inspired my own research, but also wide ranges of perspectives on AI. As their day one assignment, each of my students also had to read one portfolio from a Dawson professor that preceded me in the AI COP. Students interested in ethical questions were directed to Robert Stephens Dawson page; those interested in the Economics of AI were sent to Ahmad Banki’s page; and so on.
The level of AI literacy of students at the beginning of the course was impressive. Just a few years ago, AI was still portrayed in conversations as this transcendent panacea that would solve all sorts of problems, from global warming to economic inequalities. Today, students are aware of both the potential and risks associated with such powerful tools. Zoomers (or Gen Z’s, if you prefer) are particularly aware of bias and data privacy dimensions.
To help students leverage this background knowledge into sets of questions and a university level paper, I added in folders a short description to introduce each theme/field. You can look at them as pitches as to why, out of all the AI related subject, one specific AI subset of questions is worth dedicating the biggest project of your CÉGEP social science student career.
Aside from thematic descriptions, the Moodle Folders included a list of resources for each themes and fields. Each resource is present in only one document, but there are some thematic and fields overlaps. Note that on some occasions, and only when the documents were available online without a firewall, I shared with students PDF documents with highlights and comments about how the resource related to our class or my own research.
Moodle Folders: Thematic Description and Condensed List of Resources
The four themes the course explores are:
The three fields explored are:
You can find all seven descriptions from the Moodle Folders here. Note that at the beginning of the semester, the Moodle folders included only one or two preselected web resources, as I did not want to drown them in material early in the research process. Also note that for the three fields, I make connections with the themes. This is because students were asked to select a combination of one theme and one field, to better orient their research.
Thematic Subgroups & Student Papers
Once students selected subjects for their IS paper, they were divided in three thematic subgroups. The division of students in subgroups helped with the central coaching dimension of IS. Students consistently teamed up with members of their groups to complete labs, share resources during mini-oral presentations early in the semester, peer-review projects with similar theses, and so on. Being teamed up with colleagues that are working on similar topicd optimized these learning exercises. The three subgroups were as followed:
- ethics & healthcare
- economy & regulation
- law enforcement
The law enforcement group was the most populated, which is not that surprising giving this was a Law, Justice, and Society IS. My own expertise in Algorithmic Policing, which is the subject of my PhD dissertation, perhaps additionally tilted the balance here. In any cases, students had to relate their project in some way to liberal democracies, the background theme of this class. The theme of liberal democracy avoided students to get too sidetracked in their project, and to think of the social impact of AI in terms of democratic practices, polarization, power, etc. Still, as this was an IS seminar, students had the flexibility to choose other related AI questions. Most stuck to the suggested options, but there were interesting contributions in the other fields such as Education, Cyberwarfare, and Transport.
Here is a selection of titles from the comprehensive assessment produced by my students, during the Winter 2022 semester (IS Section 22). I included the names of the students that authored these fine papers (with their written informed consent) and abridged some of the titles:
- Emotional Intelligence: AI, The Modern Frankenstein – Sasha Lamontagne
- The Danger of Artificial Intelligence in the Military – Metaxia Giotsalitis
- Artificial Intelligence as a Utopian Key for Education – Naba Qayum
- Policing & AI: The Precision of Bias – Zachary Honsinger-Ruoff
- The Doomed Future of Healthcare Systems – Katherine Colacone
- Regulating GAFAM: Individual Privacy, Economic Domination, and Political Influence – Kamil Kowalski
- Mathematical Discrimination: How AI is Used Against Disadvantaged Groups of People – Saarah Jabar
- Facial Recognition: The Imperfect Future of Policing – Sabrina Gonzalez Roque
- The Politics of Digital Democracy – Charlotte Pelletier-Burke
Thesis Statements Lab: Understanding that AI is Socially Constructed
Labs are an integral component of the Integrative Seminar course. Early in the semester, students were teamed up in groups of three to complete a Lab titled “Sociotechnical Imaginaries Thesis Statement Lab”. This is the first lab students completed after the initial exercise where students are asked to search for peer-reviewed sources in three disciplines (which most professors assigned in some shape or form). The goal of this follow-up lab was to help students better appreciate and evaluate the references they had gathered. Artificial intelligence is socially constructed. Different actors actively try and promote their own vision of what AI is, but also what it means for the future of society. Janet Chan refers to these purposive perspectives as “sociotechnical imaginaries,” which are “collectively held, institutionally stabilized, and publicly performed visions of desirable futures, animated by shared understandings of forms of social life and social order attainable through, and supportive of, advances in science and technology” (Jassanof 2015, 4 from Chan 2021).
Chan’s book chapter inspired this lab, and the lab PPT summarized the four perspectives she describes:
- the utopian view
- the civil rights view
- the social science view
- the data scientist view
The lab started with introducing the concept of sociotechnical imaginary and the four AI imaginaries. Students were asked to categorize the peer-reviewed sources they collected into these four perspectives. They were then tasked, in teams of three, to propose thesis statements on their own topics from these same imaginaries (one thesis from the point of view of a data scientist, one from the point of view of a civil rights activist, and so on). From a methods perspective, this served as a good refresher of RM lessons on how to write efficient thesis statements. The lab also allowed students to critically assess the sources they had found in the previous class. This reminded them that a source being peer-reviewed does not mean it is not animated by a particular social construction of technology, intentional or not.
Integrative Seminar: French Component
The mandatory French component of this class consisted in finding two sources (including one peer-reviewed) in French. There is a lot of AI-related content in French, so this was not too challenging for students. Most students ended up using these references in their comprehensive assessments. This allowed them to consult material with at times distinct ontological and epistemological perspectives on AI.
In addition, much of the resources found by students in French were produced by local scholars. On this point, it is not an accident if there is such an emphasis on AI in our institution. Dawson College is located in Montreal, and is part of a rich AI ecosystem (see a partial cartography). I therefore used the lab to introduce to the students the different actors that compose this universe, and introduced Montreal-based AI startups, research groups, apps, or declarations that have had global impacts. We also discussed Dawson AI, as well as perspectives for their futures if they wanted to pursue a career in this field, in Montreal or elsewhere. The last semester of your CÉGEP career is both stressful and exciting; decisions you make at this crucial turning point might follow you your entire life. To help with this process, I shared with them the podcast and website 80 000 hours that some students found helpful when thinking about their career possibilities.
Tutorials
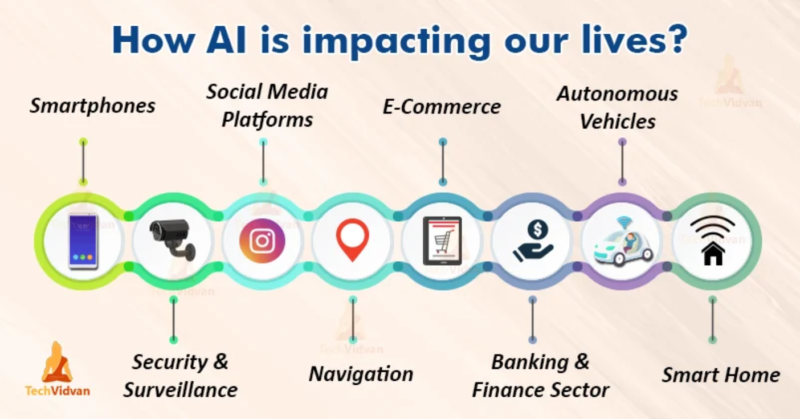
To gage the level of AI literacy of my group, I had students complete short tutorials on AI during the first week of class. I assigned different tutorials at random and asked for student feedback afterwards. The one they preferred was the Techvidan AI Tutorial. The interface is user-friendly, and CÉGEP student accessible. One thing to know is that it emphasizes healthcare dimensions, which in my case worked well as most of my examples were in policing. In terms of content, the Techvidan Tutorial is a good starting point as it does not cover too much technical material that might scare some social science students. (It is probably wiser to start with the social impact of technology and figure out Bayesian mathematics latter.)
During the semester, students at times expressed difficulties understanding technical concepts they read about in their peer-reviewed resources. Indeed, many social impact of AI scholars tend to assume concepts such as deep learning and machine learning are common knowledge. I sent them here for a concise survey of definitions (that regularly gets updated), unless they focused on the economy (The McKinsey Global Survey on the State of A.I., 2021). There were a few students who requested to go even deeper.
Other materials to prepare an AI focused integrative seminar:
Lab: Practicing with MLA & Algorithmic Policing
Class 2 and 3 PPT slides
Research Methods course introducing Artificial Intelligence themes
Predictive Policing
RM is the first of three mandatory methods courses for social science students at Dawson. A useful tip I got from a fellow RM professor to raise students’ interest in methods was using research that influences my own work. The logic is if the instructor is excited by a specific research project, students will too. Despite my efforts, research on Automatic Licence Plate Readers did not particularly spark students’ interest. However, one example I found rose students’ curiosity was the study of Predictive Policing (PP). Discussed cases included Vancouver, New Orleans, Chicago (person-based, see Saunders et al. for more details), Shreveport, and Los Angeles, on which we focused.
PP allowed good discussions on the nature of data, as well as problematic correlations that police officers make based on such AI. After introducing the technology, I had students do an exercise based on the LAPD’s Palantir Chronic Offenders Checklist. Students notably had to list assumptions and other problematic methodological dimensions of this document.
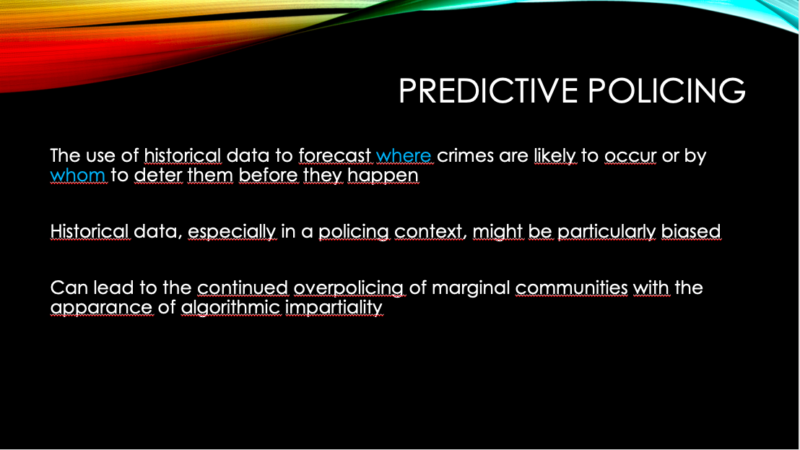
LA was likewise an interesting case for a RM lesson as this case saw social scientists allied with civil society and journalists to concretely change police AI policy, namely the abandonment of problematic PredPol software by the LAPD. On this, a report by the civil society group Stop LAPD Spying coalition can be found here, I discussed its methods and biases in my class. The Intercept also did some great reporting (among many journalists who worked on this). The LAPD police commission report is also worth a look to examine the absence of accountability mechanisms to early police AI adoption.
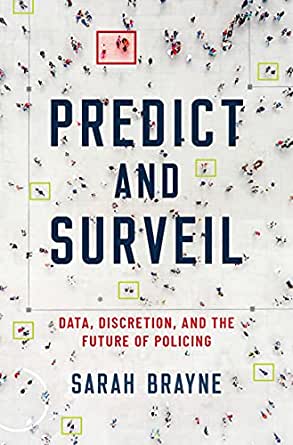
From the social scientist side, the work of Canadian sociologist Sarah Brayne is worth a look, both her book and article. I had RM students read this short piece (How the LAPD and Palantir Use Data to Jusitify Racist Policing), which contextualizes her book and its impact. Brayne’ work is useful as it connects most of the elements I wanted to discuss in terms of introducing big data policing and predictive policing to my students. In addition, it allowed me to introduce RM concepts of ethnographic research, field work, and in-depth interviews.
Ted Talk Introducing Data
To help illustrate my introduction lecture on quantitative and qualitative data, my students and I watched this Ted talk by Amy Webb, titled “How I Hacked Online Dating.” Students then had to answer a few questions on the talk; i.e what is an algorithm, name a qualitative/quantitative variable used by the speaker, etc. I found this was a light yet instructive complement to help students understand different types of variables. Webb also does a great job at efficiently vulgarizing the concepts of algorithms and data. If you enjoy her talk, I strongly recommend her book The Big Nine.
Automatic Transcription Services
Part of what I try to share with my students throughout my RM classes is that social science research is an evolving art. The range of human experiences that we explore is constantly growing, and with that the techniques we use to perfect our research processes. Technology too has a role to play in this. Think, for instance, of advances in the fields of voting behaviour made possible through powerful computers in the past 30 years. Most examples that come to mind when thinking of how technology (or even AI) has transformed social sciences in the past decades are intuitively linked to quantitative methods, and math-y fields such as economics or inference-based sociology. Yet, AI has already revolutionized the way qualitative methods are conducted. Technological advances create huge opportunities for research, especially since time constrains have long been a huge hurdle for quality qualitative research.
My own research experience has taught me the interview process is a prime example of this. For my master’s degree, I had to fly from Paris to the Dominican Republic twice, conduct interviews in person, transcribe them by hand, and translate them. In this entire experience, the most aggravating part was the process of transcribing interviews, which often took four to five times longer than the actual interviews. In contrast, by the time I was a PhD candidate, I conducted most interviews via Zoom (#2020) and used an automatic transcription software to transcribe them. I was able to interview someone in New York at 9AM, another in Vancouver at 2PM, and transcribe both conversations the same day. I decided to use this experience to demonstrate to my RM classes how advances in the field of AI can be used to improve our time management as researchers. I assume most readers are comfortable with the Zoom part, which is why I emphasize here automatic transcription software, a mature AI technology that can help researchers and RM instructors today.
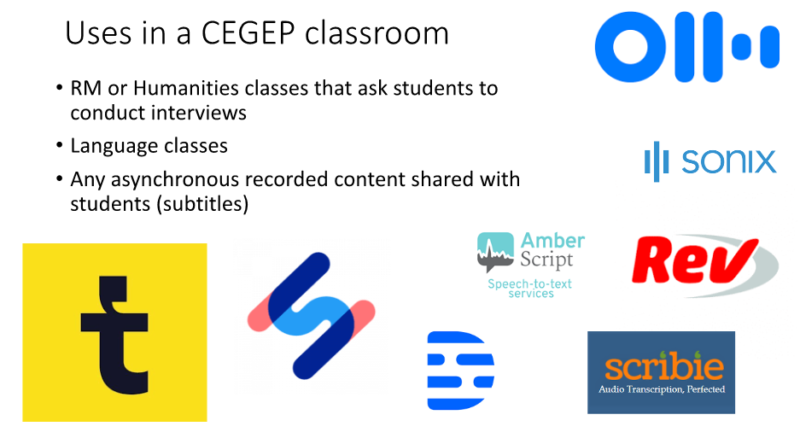
The main project for my RM students is to go through the entire process of preparing an interview, including desk research, building a consent form, developing an interview schedule, recording the interview, transcription, and how to leverage the data from the interview. During the first lab, I have students interview themselves for five minutes, and then transcribe these by hand. The lab took over 45 minutes. Then, I have them transcribe this same conversation using an automatic transcription software. The lesson, albeit it might seem obvious: there is no reason to spend hours transcribing interviews when you could be spending this time analyzing the data.
This being said, it is important to underline to the students that they should still verify the exactitude of the transcript and make the corrections if the software made errors. Also note that most software require payment, but many have one hour trial deals that do not require a credit card number. One that has continuously offered this deal is sonix.ai, which many of my students used. As prices and offers fluctuate, it will require a verification of the different software offers prior to suggesting it to your students. During a PED Day presentation in October 2021, I talked more in depth about choosing the right software for CÉGEP students, list about a dozen options, and discuss ethical consideration to share with them. The Ped Day presentation and supporting material can be found here, and here are my PPT slides on it.